The landscape of Language Model (LM) development has entered an era of intense competition, with notable players like Google, OpenAI, and Meta Llama (formerly Facebook). These technological juggernauts are spearheading the race to create cutting-edge Language Models, each striving to push the boundaries of natural language understanding and generation.
Google, renowned for its prowess in search engines and AI-driven applications, has ventured into this domain with Google Gemini, aiming to create a versatile and powerful LM capable of handling various complex tasks. OpenAI, on the other hand, has gained prominence with its GPT (Generative Pre-trained Transformer) series, notably ChatGPT, which has revolutionized conversational AI with its sophistication and adaptability. Additionally, Meta Llama, part of the Meta (formerly Facebook) family, has been actively contributing to the LM market, striving to innovate and advance language technologies within its ecosystem.
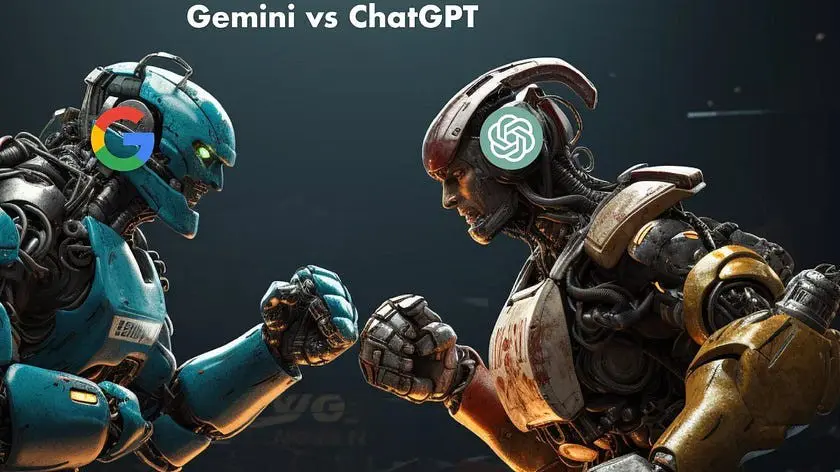
As these tech giants continue to invest resources, talent, and innovation into their respective language models, the competition intensifies, promising groundbreaking advancements that could reshape how we interact with technology, automate tasks, and comprehend natural language. This race between Google, OpenAI, and Meta Llama not only fuels innovation but also drives the rapid evolution of language models, bringing us closer to achieving more sophisticated and human-like AI capabilities.
Please note that this article does not aim to directly compare the capabilities of various products. Instead, it focuses on elucidating the distinct Language Model (LLM) solutions offered by the two companies and their comparative pricing. It’s important to clarify that this article does not serve as a performance evaluation between the solutions.
The recent advancements in generative AI have sparked a competitive landscape, with Google and OpenAI at the forefront, each offering cutting-edge models. Google’s Gemini and OpenAI’s ChatGPT-4 are among the most powerful AI tools available, and their features and pricing models have been the subject of much discussion. In this blog, we will delve into the key features of these two models and explore the pricing strategies employed by Google and OpenAI.
Google Gemini
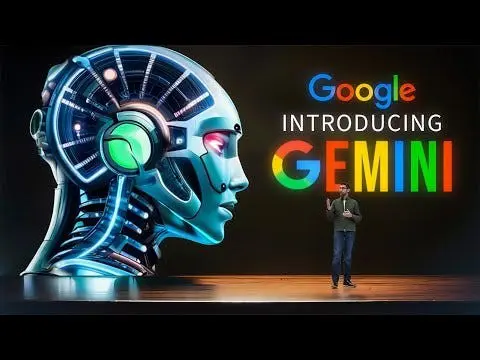
Google recently unveiled Gemini, its latest AI model that has garnered significant attention in the industry. Here are some key features of Google Gemini:
Google Gemini represents a cutting-edge artificial intelligence model designed by Google, demonstrating proficiency not only in text comprehension but also in interpreting images, videos, and audio inputs. Functioning as a multimodal model, Gemini boasts the ability to perform intricate tasks in domains such as mathematics, physics, and other disciplines. Additionally, it showcases proficiency in comprehending and generating high-quality code across diverse programming languages.
Presently, Gemini is accessible through integrations with Google Bard and the Google Pixel 8. Over time, it is expected to seamlessly integrate into various other Google services.
- Multimodal Capabilities: Gemini is a multimodal AI model, capable of processing text, images, audio, and video simultaneously, giving it a broad range of applications.
- Three Versions: Gemini is available in three versions: Ultra, Pro, and Nano, each catering to different use cases and performance requirements.
- Performance: Google executives have claimed that Gemini outperforms OpenAI’s GPT-3.5, showcasing its high capabilities.
- Deployment: Google plans to license Gemini to customers via Google Cloud, allowing them to integrate the model into their applications[4].
OpenAI’s ChatGPT-4
OpenAI’s ChatGPT-4 is a powerful language model known for its advanced natural language processing capabilities. Here are some key features of ChatGPT-4:
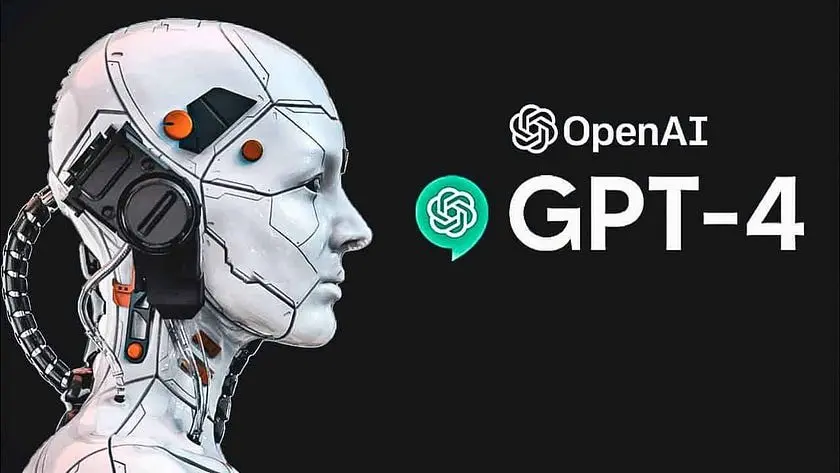
- Language Model: ChatGPT-4 excels in generating and understanding text, making it suitable for a wide range of natural language processing tasks.
- Real-world Applications: It has extensive real-world applications, including virtual assistants, educational tools, information retrieval, and task automation.
- Powerful: ChatGPT-4 is noted for being more powerful than existing models, and it has been benchmarked against other models in the field.
Pricing Strategies
The pricing strategies of Google and OpenAI for their generative AI models have also been a point of interest. Google’s character-based billing model has been noted to be advantageous for certain language speakers, while OpenAI’s token-based approach appears to favor English speakers. It’s essential for businesses to carefully evaluate their specific requirements, considering factors beyond pricing alone, such as the capabilities of the models, integration with existing infrastructure, and long-term strategic objectives.
Both Google’s Gemini and OpenAI’s ChatGPT-4 represent significant advancements in the field of generative AI. The choice between the two would depend on the specific requirements of the application or task at hand, as well as the pricing considerations for different language speakers. As the generative AI landscape continues to evolve, it will be fascinating to witness the further developments and innovations from these leading AI providers.
Language inequality
Examining the billing differences between OpenAI and Google prompts an exploration into whether OpenAI’s tokenizers exhibit bias towards the English language and how Google’s character approach differs fundamentally.
Illustrated by red dots representing English within each billing model and blue dots depicting 49 other languages in the dataset, it’s evident that OpenAI’s tokenizers — specifically cl100k used by ChatGPT and AdaV2 for embedding, alongside p50k utilized by the Text models and other embeddings models — demonstrated a noticeable bias towards English. This aligns with expectations, considering that much of the internet content is in English. Notably, the p50k tokenizer charges nearly 16 times more for Malayalam, one of the four languages spoken in South India, compared to English.
A noteworthy observation is that English emerges as the least expensive language for both of OpenAI’s tokenizers. Conversely, it holds a middle ground for Google’s character counter, implying that certain languages might find greater cost-efficiency through Google’s solution. However, it’s crucial to consider the broader implications of pricing rather than solely focusing on tokenization biases.
Text and chat
Navigating the comparison between OpenAI and Google becomes nuanced when evaluating their offerings in text and chat models.
In terms of text-specialized models, OpenAI provides four distinct variants, ranging from the high-performing DaVinci to the lighter Ada. However, DaVinci’s significantly higher cost places it in a separate category. Text_Bison aligns closely with OpenAI’s Curie model in terms of pricing, while Babbage and Ada exhibit minimal pricing differences owing to their narrow variance of 0.0001$ per thousand tokens. To discern substantial pricing disparities, significantly extensive text input would be required.
An important aspect to consider is the model comparison suggested by Google, proposing that Text_Bison is most akin to… DaVinci. This alignment implies that despite its position, Bison’s conception and performance mirror that of DaVinci. If validated, this comparison would signify a considerable advantage for Google concerning the price-to-performance ratio.
Moving to chat models, OpenAI offers four Chat-GPT variants, each with varying context sizes for generating responses. The logical comparison for Chat-Bison is with GPT3.5 in its 4K context version, as they are deemed nearly equivalent in capabilities and can process a context composed of up to 4096 tokens.
A noteworthy aspect of Chat models is OpenAI’s differential pricing for input and output tokens. This means that generating text incurs a different cost than providing text and requesting a summary of it, creating varied billing structures based on the type of task performed.
Analyzing these Chat-models comparisons highlights some intriguing findings:
– For languages like Korean or Japanese, Google emerges as the more economical option.
– In languages such as Spanish, French, or German, the cost-effective choice hinges on specific requirements: generating extensive text with a relatively brief prompt or summarizing substantial text volumes.
Data Used
To facilitate a comprehensive comparison of various models and languages, I opted to not only examine them theoretically but also practically. For this purpose, I sought a dataset that would enable the comparison of sentences conveying the same meaning across different languages.
The dataset I utilized is called “MASSIVE,” provided by Amazon. This dataset comprises sentences captured by the Alexa assistant, encompassing 50 languages. However, I narrowed down my focus to eight languages — English, French, German, Spanish, Italian, Portuguese, Korean, and Japanese. I selected these languages arbitrarily, aiming for diversity to cover potential differences.
In each language subset, all sentences were consolidated into a single cohesive text. This consolidated text conveys identical meanings across languages, incorporating numerous sentences. This approach was adopted to assess the models’ performance and unveil pricing disparities in a standardized manner.
Google’s Gemini and GPT-4 by OpenAI are cutting-edge advancements in the realm of AI, each emphasizing distinct visions and functionalities:
GEMINI’s Vision and Purpose:
GEMINI sets its sights on democratizing AI worldwide, striving to create inclusive opportunities and drive innovation that fosters economic progress through AI technologies.
GPT-4’s Vision and Purpose:
GPT-4 focuses on enhancing safety and utility, aspiring to develop more sophisticated language models with improved creativity and problem-solving capabilities.
Multimodality:
- GEMINI: This versatile model excels in multimodal tasks by comprehending and amalgamating various data types, including text, code, audio, images, and videos. It is adaptable across different sizes, ranging from Ultra to Nano.
- GPT–4: GPT-4 introduces visual comprehension, allowing it to process visual information and generate responses based on this input.
Performance:
- GEMINI: Gemini Ultra, the flagship model, surpasses benchmarks in language understanding and multimodal tasks, showcasing superior performance across diverse domains.
- GPT-4: GPT-4 demonstrates marked improvements over its predecessor, particularly in problem-solving accuracy and performance on standardized tests, such as the Uniform Bar Exam and Biology Olympiad.
Reasoning Abilities:
- GEMINI: Gemini 1.0 showcases advanced multimodal reasoning capabilities, extracting insights from complex written and visual data, particularly excelling in disciplines like mathematics, physics, and coding.
- GPT-4: GPT-4 outperforms its predecessor in sophisticated reasoning tasks, exemplified by its adeptness in scheduling meetings based on the availability of multiple participants.
Safety and Alignment:
- GEMINI: Gemini undergoes rigorous safety evaluations, encompassing bias and toxicity analysis. The model engages in thorough testing and collaboration with external experts to identify and mitigate potential risks.
- GPT-4: GPT-4 showcases safety enhancements, reducing the likelihood of responding to disallowed content requests by 82% and increasing factual response production by 40% compared to GPT-3.5. OpenAI continuously refines the model based on real-world usage and user feedback.
Applications and Partnerships:
- GEMINI: Google plans to integrate GEMINI into its products like Bard and Pixel, augmenting their reasoning, planning, and writing capabilities. Additionally, GEMINI is available to developers and enterprise customers through the Gemini API.
- GPT-4: GPT-4 collaborates with various organizations, including Microsoft Bing, Duolingo, Stripe, and Morgan Stanley, exploring the potential of advanced language models in diverse domains such as language learning, accessibility, user experience, and knowledge management.
How can you access Gemini?
Gemini is now available on Google products in its Nano and Pro sizes, like the Pixel 8 phone and Bard chatbot, respectively. Google plans to integrate Gemini over time into its Search, Ads, Chrome, and other services.
Also: I asked DALL-E 3 to create a portrait of every US state, and the results were gloriously strange
See what the creators say about Gemini: https://youtu.be/jV1vkHv4zq8
Developers and enterprise customers will be able to access Gemini Pro via the Gemini API in Google’s AI Studio and Google Cloud Vertex AI starting on December 13. Android developers will have access to Gemini Nano via AICore, which will be available on an early preview basis.
Are there different versions of Gemini?
Google describes Gemini as a flexible model that is capable of running on everything from Google’s data centers to mobile devices. To achieve this scalability, Gemini is being released in three sizes: Gemini Nano, Gemini Pro, and Gemini Ultra.
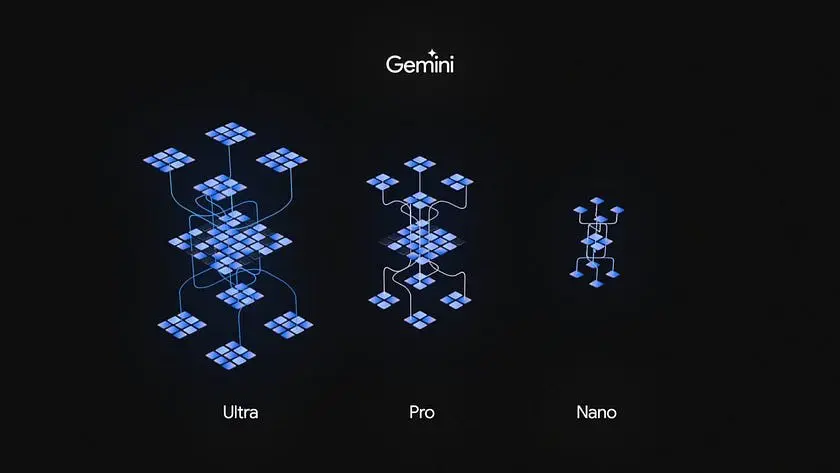
- Gemini Nano: The Gemini Nano model size is designed to run on smartphones, specifically the Google Pixel 8. It’s built to perform on-device tasks that require efficient AI processing without connecting to external servers, such as suggesting replies within chat applications or summarizing text.
- Gemini Pro: Running on Google’s data centers, Gemini Pro is designed to power the latest version of the company’s AI chatbot, Bard. It’s capable of delivering fast response times and understanding complex queries.
- Gemini Ultra: Though still unavailable for widespread use, Google describes Gemini Ultra as its most capable model, exceeding “current state-of-the-art results on 30 of the 32 widely-used academic benchmarks used in large language model (LLM) research and development.” It’s designed for highly complex tasks and is set to be released after finishing its current phase of testing.
The comparative analysis of GEMINI by Google and GPT-4 by OpenAI illustrates remarkable advancements in AI technology. GEMINI’s focus on multimodality and performance stands in contrast to GPT-4’s emphasis on safety, alignment, and creative problem-solving. Both models exhibit enhanced reasoning capabilities and real-world applications through strategic partnerships, signaling the promising trajectory of AI evolution.
As the AI landscape progresses, addressing limitations like biases and adversarial prompts remains imperative. Transparency and user education are pivotal in guiding the development of these models toward responsible and ethical AI applications.
Looking ahead, the anticipated innovations in the AI space in the coming years promise even greater advancements. Exploring how these models evolve and witnessing their global utilization presents exciting prospects for the future.
Please Refer to Introducing Gemini: (blog.google) and GPT-4 (openai.com) for comprehensive details on these models.
Dive into the captivating world of language and embark on an adventure of possibilities.
Disclaimer:
The information provided herein is based on available documentation at the time of writing. For the most current and accurate details, please refer to the official documentation of GEMINI and GPT-4. When considering choices between GEMINI by Google and GPT-4 by OpenAI, businesses should carefully assess their specific needs, weighing factors beyond pricing alone. Evaluating model capabilities, integration possibilities, and long-term strategic objectives is crucial in navigating the rapidly evolving landscape of generative AI.
Citations:
[1] https://medium.com/google-cloud/generative-ai-pricing-openai-vs-google-cloud-8fe708a5636a
[2] https://www.cnbc.com/2023/12/07/google-shares-pop-after-company-announces-gemini-ai-model.html
[3] https://www.pymnts.com/news/artificial-intelligence/2023/ai-wars-googles-gemini-cant-stop-comparing-itself-to-openai/
[4] https://www.hindustantimes.com/technology/google-gemini-vs-openais-chatgpt-comparing-the-two-most-powerful-generative-ai-tools-101701883876150.html
[5] https://www.businessinsider.com/google-gemini-ai-performance-openai-chatgpt-gpt4-2023-12