In the era of rapid digital transformation, social media has become a vital platform for businesses and individuals to interact, share information, and stay connected. The sheer volume of content generated on social media platforms poses a significant challenge for effective monitoring and response. Enter Retrieval-Augmented Generation (RAG) – a groundbreaking AI approach that promises to revolutionize how we monitor social media and generate responses. In this blog, we will delve into what RAG is, how it works, and its transformative potential in the realm of social media
Understanding Retrieval-Augmented Generation (RAG)
RAG is an advanced AI model that combines the strengths of retrieval-based and generative models. Traditional retrieval-based models search a database of documents to find relevant information, while generative models create new content based on the input they receive. RAG harnesses the power of both by first retrieving relevant information and then generating contextually appropriate responses using that information.
At its core, RAG consists of two main components:
- Retriever: This component searches a large corpus of documents (e.g., social media posts, articles, FAQs) to find relevant information based on the input query.
- Generator: Using the retrieved information, this component generates a coherent and contextually relevant response.
This dual approach allows RAG to provide more accurate and informative responses compared to traditional models that rely solely on pre-defined responses or purely generative methods.
The Role of RAG in Social Media Monitoring
Social media monitoring involves tracking and analyzing social media channels for mentions of a brand, competitors, products, or any relevant keywords. The goal is to understand public sentiment, identify emerging trends, and engage with the audience effectively. RAG can significantly enhance this process through:
- Real-Time Insights: By continuously scanning social media platforms, RAG can retrieve the latest posts and comments relevant to a specific query. This ensures that businesses have access to real-time insights, allowing them to respond promptly to emerging issues or trends.
- Enhanced Sentiment Analysis: RAG can retrieve contextual information around social media mentions, providing a deeper understanding of the sentiment behind posts. This enables more nuanced sentiment analysis, helping businesses gauge public opinion more accurately.
- Crisis Management: In the event of a PR crisis, timely and accurate responses are crucial. RAG can quickly retrieve relevant information and generate appropriate responses, helping to mitigate the impact of negative publicity.
- Competitive Analysis: Monitoring competitors’ social media activities is essential for staying ahead in the market. RAG can retrieve and analyze competitors’ posts, providing valuable insights into their strategies and audience engagement.
Response Generation with RAG
Responding to social media monitoring interactions promptly and appropriately is key to maintaining a positive brand image and fostering customer loyalty. RAG can enhance response generation in several ways:
- Personalized Responses: RAG can retrieve information about previous interactions with a customer and generate personalized responses that acknowledge their history and preferences. This level of personalization can significantly enhance customer satisfaction.
- Consistent Messaging: By retrieving relevant guidelines and previous responses, RAG ensures that all interactions are consistent with the brand’s voice and messaging. This consistency is crucial for maintaining a cohesive brand image.
- Automated yet Human-like: While automation can streamline social media management, maintaining a human touch is important. RAG’s ability to generate contextually relevant and coherent responses makes automated interactions feel more natural and human-like.
- Scalability: For businesses with a large social media presence, manually responding to every interaction is impractical. RAG enables scalable response generation, ensuring that all customer queries and comments are addressed promptly.
Case Studies and Applications
Case Study 1: Customer Support
A leading e-commerce company implemented RAG for their social media customer support. By integrating RAG with their CRM system, the company was able to retrieve customer purchase history and generate personalized responses. This resulted in a 30% reduction in response time and a 20% increase in customer satisfaction scores.
Case Study 2: Brand Monitoring
A global fashion brand used RAG to monitor social media for mentions of their products and competitors. The real-time insights provided by RAG allowed the brand to identify and capitalize on emerging fashion trends, leading to a 15% increase in sales during the following quarter.
Challenges and Future Directions
While RAG offers numerous benefits, there are challenges to consider:
- Data Privacy: Retrieving and using customer data for generating responses raises privacy concerns. Ensuring compliance with data protection regulations is paramount.
- Quality of Retrieved Data: The effectiveness of RAG depends on the quality of the retrieved data. Poor-quality data can lead to inaccurate or irrelevant responses.
- Computational Resources: Implementing RAG requires significant computational resources, which can be a barrier for smaller businesses.
Despite these challenges, the future of RAG in social media monitoring and response generation looks promising. Advancements in AI and machine learning will continue to enhance the capabilities of RAG, making it an indispensable tool for businesses looking to optimize their social media strategies.
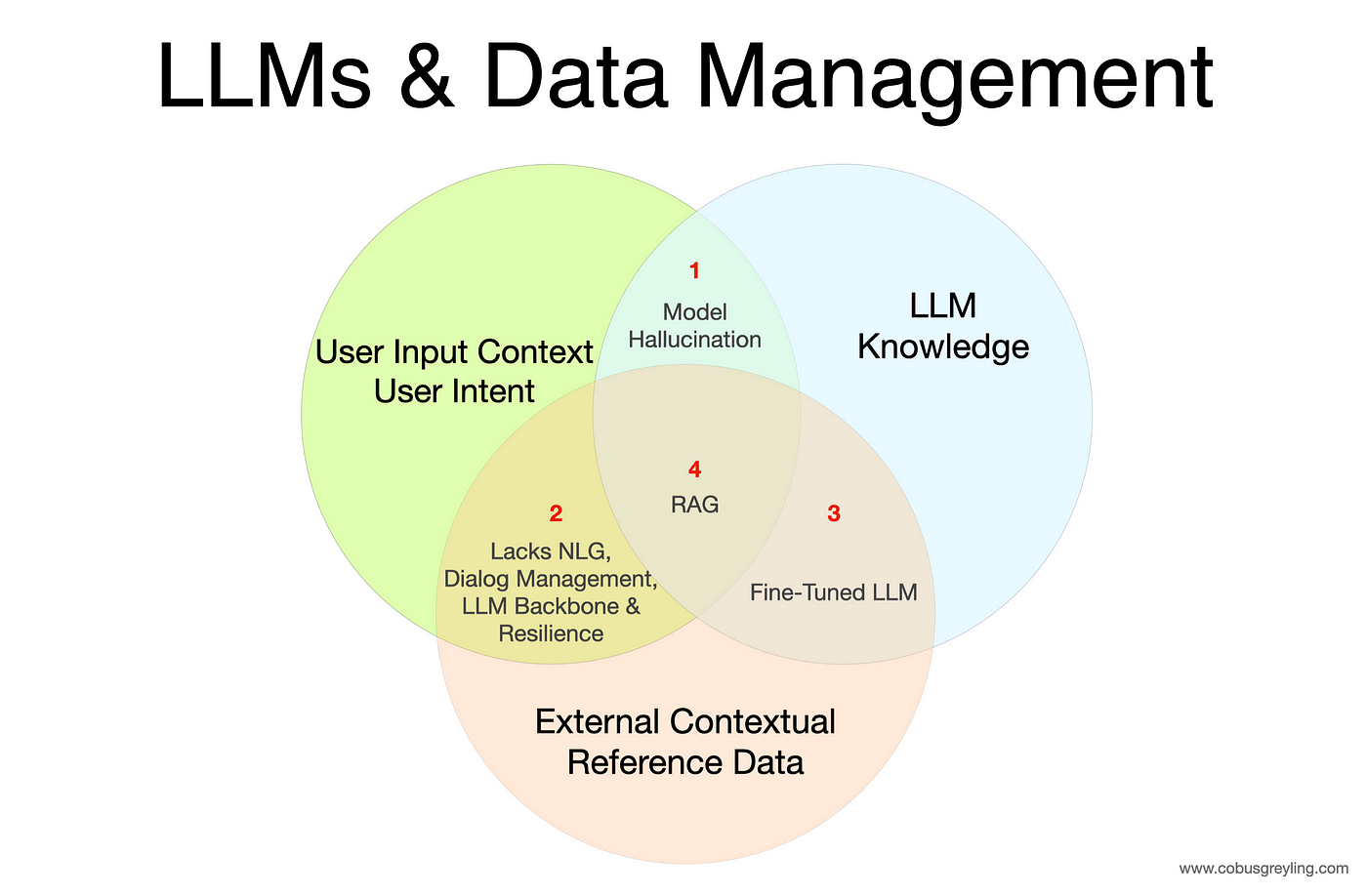
Retrieval-Augmented Generation represents a significant leap forward in the field of social media monitoring and response generation. By combining the strengths of retrieval-based and generative models, RAG offers real-time insights, enhanced sentiment analysis, personalized responses, and scalable solutions. As businesses continue to navigate the dynamic landscape of social media, leveraging RAG can provide a competitive edge and foster deeper connections with their audience. The future of social media management is here, and it is augmented by the power of retrieval and generation.