In recent years, Generative Adversarial Networks (GANs) have revolutionized the field of image synthesis, showcasing unprecedented capabilities in generating realistic images. This blog delves into the mechanisms behind GANs, their practical applications, and the challenges and future directions of this groundbreaking technology.
Understanding Generative Adversarial Networks (GANs)
Generative Adversarial Networks (GANs) consist of two neural networks—the generator and the discriminator—engaged in a continuous, adversarial process:
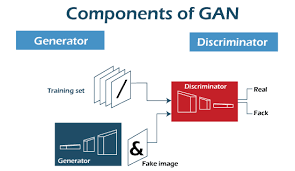
Generator: Creates synthetic data samples (e.g., images) from random noise, aiming to produce samples indistinguishable from real data.
Discriminator: Evaluates the authenticity of the samples, distinguishing between real and generated data.
Adversarial Training: The generator improves its samples to fool the discriminator, while the discriminator enhances its ability to detect fakes, driving both networks to excel over time.
How GANs Work?
The GAN training process involves several steps:
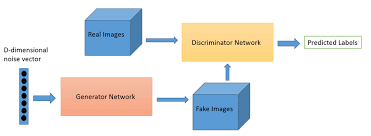
Initialization: Randomly initialize the weights of both the generator and discriminator.
Training Loop:
- Generator Training: Generates a synthetic sample and passes it to the discriminator.
- Discriminator Training: Classifies the sample and computes a loss based on its prediction accuracy.
- Loss Computation: Calculates the generator’s loss based on its ability to fool the discriminator and the discriminator’s loss on classification accuracy.
- Weight Update: Adjusts the weights of both networks to minimize their respective losses.
Iteration: Repeats the process iteratively until the generator produces highly realistic samples.
Applications of GANs in Image Synthesis
1. Image Generation:
GANs can create high-quality, realistic images from scratch, widely used in art, product design, and virtual environments for games and movies.
2. Image-to-Image Translation:
GANs transform images from one domain to another, such as converting sketches to detailed images or day images to night scenes. Key frameworks include:
- Pix2Pix: Translates edge maps to photos.
- CycleGAN: Performs translation between unaligned datasets.
3. Super-Resolution:
GANs enhance low-quality images’ resolution, valuable in medical imaging, satellite imagery, and photo restoration.
4. Style Transfer:
GANs apply artistic styles from one image to another, enabling unique and visually appealing artworks.
5. Data Augmentation:
GANs generate additional training data, improving models’ performance in tasks like image recognition and object detection.
6. Deepfake Creation:
While controversial, GANs create realistic fake images and videos, used in entertainment, film production, and virtual reality.
Impact of GANs on Various Industries
1. Entertainment and Media:
GANs revolutionize special effects, virtual characters, and immersive environments in movies and games, enhancing content generation for social media and advertising.
2. Healthcare:
GANs enhance medical imaging, aiding diagnosis and treatment. They generate synthetic medical images for research and training, improving data quality and accessibility.
3. Fashion and Design:
GANs generate new clothing patterns, virtual try-ons, and product prototypes, helping designers visualize and create innovative products efficiently.
4. Art and Creativity:
GANs enable artists to explore new creative possibilities, producing unique artworks and music compositions through human-AI collaboration.
5. Security and Surveillance:
GANs enhance security by generating synthetic data for training surveillance systems and improving facial recognition technologies.
Challenges and Future Directions
1. Training Stability: GANs require careful tuning of hyperparameters and balancing the generator and discriminator.
2. Mode Collapse: Generators may produce limited data variations, failing to capture the training dataset’s diversity.
3. Ethical Concerns: GANs’ ability to create deepfakes raises ethical and legal issues.
4. Computational Resources: Training GANs is resource-intensive.
5. Future Research: Aims to improve GAN stability, output diversity, and methods to detect and mitigate GAN-generated content misuse.
Generative Adversarial Networks have revolutionized image synthesis, opening new innovation avenues across industries. As GAN technology evolves, its applications will expand, enhancing our lives and broadening human creativity’s horizons. By harnessing GANs responsibly, we can unlock a future where AI-generated images enrich various fields and drive creative possibilities.