In the rapidly evolving landscape of artificial intelligence, one of the most significant breakthroughs in recent years is the development of large language models (LLMs). These models, such as OpenAI’s GPT-3 and GPT-4, have demonstrated remarkable capabilities in understanding and generating human-like text. However, harnessing their full potential requires more than just powerful algorithms and vast amounts of data; it necessitates a nuanced approach known as prompt engineering. This blog delves into the essence of prompt engineering, its significance, and its burgeoning role in the field of generative AI.
Understanding Generative AI and LLMs
Generative AI refers to a subset of artificial intelligence that focuses on creating new content, whether it be text, images, music, or even complex designs. Large language models, like GPT-4, are at the forefront of this domain, leveraging deep learning techniques to produce coherent and contextually relevant text based on a given input, or “prompt.”
These models are trained on diverse datasets encompassing a vast array of topics, allowing them to generate text that is not only grammatically correct but also contextually appropriate. However, the quality and relevance of the output heavily depend on the nature and structure of the prompt provided.
What is Prompt Engineering?
Prompt engineering in Generative AI is the art and science of crafting prompts that elicit the desired responses from generative AI models. It involves designing and refining input queries to guide the model towards producing specific, accurate, and useful outputs. Effective prompt engineering can transform a generic response into a highly tailored and insightful one, making it a crucial skill for anyone working with LLMs.
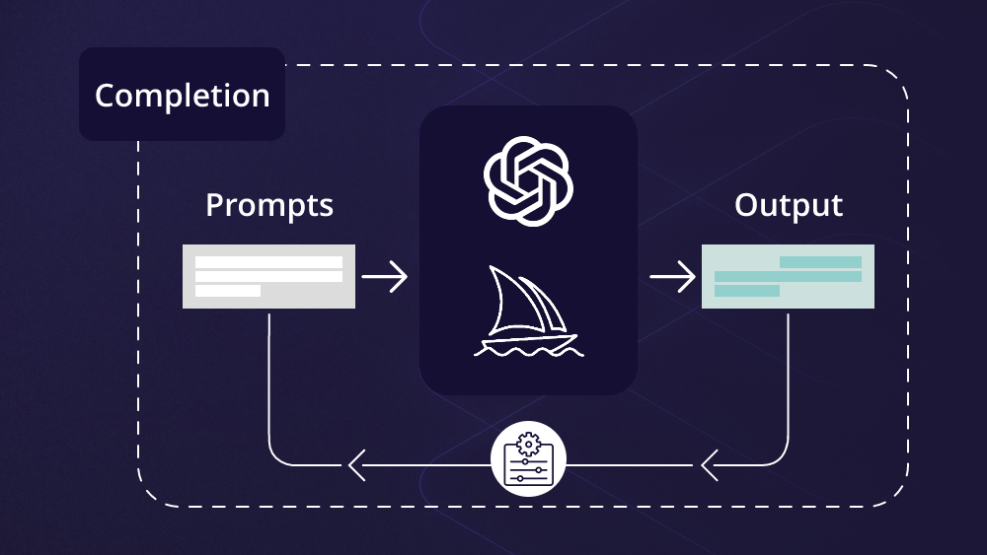
The Basics of Prompt Engineering
At its core, prompt engineering revolves around understanding how an AI model interprets input and how subtle variations in the prompt can influence the output. Here are some fundamental aspects:
- Clarity and Specificity: The prompt must be clear and specific to avoid ambiguous or irrelevant responses. Vague prompts can lead to generic outputs that may not meet the user’s needs.
- Context Provision: Providing sufficient context within the prompt helps the model understand the desired direction. This can include background information, specific instructions, or examples.
- Iterative Refinement: Prompt engineering is often an iterative process. Initial prompts may require tweaking and refinement based on the model’s responses to hone in on the desired outcome.
Examples of Prompt Engineering
To illustrate the importance of prompt engineering in generative AI, consider the following examples using a hypothetical LLM:
Example 1: Basic Prompt
- Prompt: “Explain climate change.”
- Response: “Climate change refers to long-term shifts in temperatures and weather patterns, mainly caused by human activities such as burning fossil fuels.”
Example 2: Refined Prompt
- Prompt: “Explain the primary causes of climate change and their impacts on the environment in the 21st century.”
- Response: “The primary causes of climate change in the 21st century include the burning of fossil fuels, deforestation, and industrial activities, leading to increased greenhouse gas emissions. These activities result in global warming, melting ice caps, rising sea levels, and more frequent extreme weather events.”
By refining the prompt, the response becomes more detailed and focused, demonstrating the value of prompt engineering in obtaining comprehensive and relevant information.
The Rise of Prompt Engineering
As the capabilities of LLMs have grown, so too has the recognition of prompt engineering as a critical skill. Several factors have contributed to its rise:
Enhanced Model Capabilities
The sophistication of models like GPT-4 has increased the potential applications of generative AI, from content creation and customer service to research and education. This expansion necessitates precise control over the output, which is where prompt engineering comes into play. With the ability to fine-tune responses, users can leverage these models for highly specialized tasks.
Democratization of AI
Generative AI is no longer confined to the realm of AI specialists. With user-friendly interfaces and APIs, a broader audience can now access and utilize LLMs. However, effective usage still requires an understanding of prompt engineering to maximize the value derived from these models. As a result, there is a growing demand for resources and training in prompt engineering techniques.
Diverse Applications
Prompt engineering is proving valuable across a wide range of industries. For instance:
- Healthcare: Generating patient-specific treatment plans and medical literature summaries.
- Finance: Crafting detailed financial reports and market analyses.
- Education: Developing customized learning materials and automated tutoring systems.
- Entertainment: Creating engaging content for games, stories, and interactive media.
Each of these applications benefits from precise and well-crafted prompts that guide the AI towards producing useful and contextually appropriate content.
Techniques and Best Practices in Prompt Engineering
Mastering prompt engineering in Generative AI involves understanding various techniques and best practices. Here are some key strategies:
Experimentation and Feedback
Experimentation is crucial in prompt engineering in Generative AI. Users should try different approaches, compare the results, and iteratively refine their prompts. Feedback loops, where the outputs are evaluated and used to adjust future prompts, are essential for continuous improvement.
Use of Examples
Providing examples within the prompt can help guide the model towards the desired format and content. For instance, if generating a specific type of report, including a sample report in the prompt can improve the coherence and relevance of the output.
Controlled Length
Balancing the length of the prompt is important. While too short prompts may lack necessary context, excessively long prompts can lead to information overload and reduce the model’s ability to focus on key points. Finding the right balance is key to effective prompt engineering.
Leveraging Model Settings
Many LLMs offer adjustable parameters, such as temperature and max tokens, which can influence the behavior of the model. Understanding and utilizing these settings can enhance the control over the generated content. For example, a lower temperature setting can make the model’s responses more deterministic, which is useful for tasks requiring precise and consistent output.
Prompt Templates
Developing reusable prompt templates for common tasks can streamline the process and ensure consistency. These templates can be adjusted for specific needs while maintaining a standard structure that has proven effective.
Challenges in Prompt Engineering
Despite its advantages, prompt engineering comes with its own set of challenges:
Ambiguity and Bias
LLMs can sometimes produce outputs that reflect biases present in their training data or interpret prompts in unintended ways. Addressing these issues requires careful crafting of prompts and ongoing vigilance to ensure fair and unbiased outputs.
Complexity and Scale
As tasks become more complex, designing effective prompts can become increasingly challenging. Scaling prompt engineering for large projects or across diverse domains requires robust frameworks and methodologies.
Evolution of Models
As AI models evolve, prompt engineering in Generative AI may need to be adapted. Staying current with advancements in AI and continually updating prompt strategies is essential for maintaining effectiveness.
The Future of Prompt Engineering
The field of prompt engineering is still in its infancy, and its future holds immense potential. Here are some anticipated developments:
Automated Prompt Optimization
Advancements in AI could lead to tools that assist or automate prompt engineering. These tools might analyze initial prompts, provide suggestions for improvement, and optimize them for better results, making the process more accessible to non-experts.
Integration with Other AI Technologies
Prompt engineering could become more integrated with other AI technologies, such as reinforcement learning and explainable AI. This integration would enhance the interpretability and controllability of AI models, leading to more reliable and transparent systems.
Broader Adoption and Education
As the importance of prompt engineering becomes more widely recognized, educational institutions and organizations are likely to incorporate it into their curricula and training programs. This will equip a broader audience with the skills needed to effectively utilize generative AI.
Ethical and Responsible AI
With the increasing influence of AI on society, ethical considerations will become even more critical. Prompt engineering will play a role in ensuring that AI outputs are not only accurate and relevant but also ethical and responsible. This will involve developing guidelines and best practices for crafting prompts that align with ethical standards and mitigate potential harms.
Prompt engineering is emerging as a cornerstone of generative AI, enabling users to harness the full potential of large language models. By mastering the art and science of crafting effective prompts, individuals and organizations can unlock new possibilities across various domains, from healthcare and finance to education and entertainment. As the field continues to evolve, the role of prompt engineering will only grow in significance, shaping the future of human-AI interaction and driving the next wave of innovation in artificial intelligence.