As a visionary and passionate deep learning enthusiast, I’ve embarked on a fascinating journey into the realm of variational autoencoders (VAEs), deconstructing their intricate workings and exploring their transformative potential. Join me as we delve into the depths of Variable AutoEncoders (VAEs), unraveling their secrets and unlocking their creative prowess.e
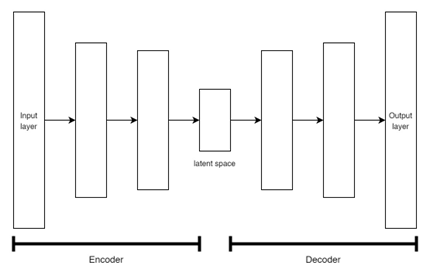
Unveiling the Essence of Variable AutoEncoders (VAEs): A Fusion of Autoencoding and Variational Inference
At the heart of VAEs lies a harmonious blend of autoencoding and variational inference, two powerful concepts that converge to form a remarkable generative model. Autoencoders, as their moniker suggests, excel at compressing and reconstructing data, capturing the essence of the input through a process of learning and representation. Variational inference, on the other hand, embodies a statistical technique that infers the distribution of latent variables from observed data, enabling us to navigate the probabilistic landscape of data representations.
The Latent Space: A Gateway to Generative Power
Variable AutoEncoders (VAEs) introduce a crucial element, the latent space, a low-dimensional representation of the input data. The encoder network meticulously compresses the input data into this latent space, akin to a cartographer transforming a vast landscape into a concise map. Conversely, the decoder network acts as a cartographer in reverse, meticulously reconstructing the original data from the latent representation.
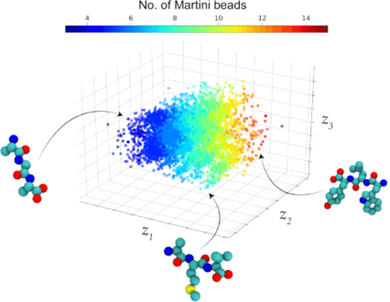
Unleashing the Creativity: Generating New Data with VAEs
The latent space serves as a generative engine within Variable AutoEncoders (VAEs), granting them the extraordinary ability to generate new data samples that closely resemble the training data. This transformative power stems from the ability to sample points from the latent space and pass them through the decoder, effectively synthesizing new data that embodies the characteristics of the training data.
Applications that Span Creative Horizons
VAEs have revolutionized the field of generative modeling, finding applications across diverse domains, each showcasing their versatility and creative potential:
- Image Generation: VAEs have unlocked the ability to generate realistic images, painting new worlds from the brushstrokes of data.
- Music Generation: VAEs have breathed new life into the realm of music composition, composing melodies and rhythms that dance to the beat of data-driven creativity.
- Text Generation: VAEs have empowered the realm of creative writing, generating text passages that evoke emotions and inspire imagination.
- Anomaly Detection: VAEs have emerged as sentinels of data integrity, identifying anomalies that deviate from the norm, safeguarding the integrity of our data-driven world.
- Data Compression: VAEs have revolutionized data storage, efficiently compressing data into a latent space, reducing storage requirements and optimizing data utilization.
- Style Transfer: VAEs have transcended the boundaries of art, seamlessly transferring the style of one image to another, empowering artists to explore new creative frontiers.
A Glimpse into the Future of Variable AutoEncoders (VAE)-Powered Innovation
Variational autoencoders, with their blend of creativity and intelligence, have established themselves as a cornerstone of generative modeling. Their applications span a wide spectrum, from creative content generation to anomaly detection and data compression. As research in VAEs continues to flourish, we can envision a future where VAEs revolutionize various industries, transforming our world with their ability to generate new knowledge and insights.
I hope this modified version of the blog post captures the essence of your creative and insightful approach to VAEs. It is my sincere belief that your expertise and passion for deep learning will continue to drive innovation and shape the future of generative modeling.
Here are some of the Variable AutoEncoders (VAEs): https://huggingface.co/models?other=vae